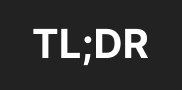
62% of corporate leaders identified a lack of talent as their biggest barrier to AI adoption
67% believe that the traditional hiring model is broken in the AI age
A new generation of tech leaders are embracing an alternate model: Blended Teams
This post is an adapted excerpt from our latest research report, the Blended Teams Playbook. Download it here.
If you're struggling to get your generative AI initiatives off the ground, you're not alone. In boardrooms across the globe, a familiar scene is playing out: executives are eager to harness the transformative power of AI, but they’re facing the same gap: Talent.
In a recent BCG study, 62% of corporate leaders identified a lack of talent as their biggest barrier to AI adoption. Even more telling? Two-thirds believe they'll need to acquire that AI talent externally rather than developing it internally.
But what if we're thinking about the talent problem all wrong?
The Perfect Storm
If you work in tech, it's been a tumultuous couple of years.
In the summer of 2022, the fundraising apocalypse hit startups. By late fall, the waves of layoffs in Big Tech began, transforming LinkedIn from a place where people extol B2B sales lessons they learned from proposing to their girlfriends to a support group for long-time employees who had been callously laid off. At the same time, Generative AI entered the chat with the launch of ChatGPT, as analysts and CEOs alike tripped over each other to project how many people the technology would replace. McKinsey estimated it would add up to $9 trillion in GDP annually. In a KPMG survey, 78% of business leaders said that generative AI would be the top emerging technology of the next 3-5 years, and 83% said they would increase their investment by more than 50% over the next 6-12 months.
Yet, the reality has failed to live up to the hype. Less than two years later, generative AI has entered the trough of disillusionment on the Gartner Hype Cycle.

It was easy to see this coming. Even though corporate leaders often declare that "failure is not an option" when launching new digital transformation initiatives, 75% fail, according to BCG research. Even that estimate might be optimistic. A separate study by McKinsey found that when you measure impact, only 16% of digital transformation efforts actually improve performance.
Exacerbating matters is that GenAI is digital transformation in hard mode. While the utility and power of GenAI are obvious to most users who spend at least ten hours experimenting with frontier models like ChatGPT4, Claude Sonnet, and Gemini, it’s trickier to identify high-ROI use cases at the organizational level. In a Gartner survey earlier this year, nearly half of global IT leaders said that their top barrier to implementing GenAI was that they could not demonstrate AI’s value and that they lacked the talent and skills within their workforce to deploy it.

From Buy to Build
GenAI is very different from previous tech revolutions because it forces you to shift from a mindset of buying software to building tech. Consider the previous tech revolutions in software:

In the first modern software revolution of the early 1990s, we lived in a Single Vendor Era: IBM, Oracle, and SAP would load software onto a mainframe that lived onsite. This software was expensive, broad, cost millions of dollars, and took months to deploy.
Then, in the early 2000s, we entered the Cloud Era, which was enabled by cloud computing and dominated by companies like Salesforce, which eliminated the need to host all of your data and software locally and onsite. They forced legacy competitors to follow suit.
Next, in the 2010s, we entered the Vertical SaaS Era, as a wave of startups rose to develop point solutions for specific industries, from fintech to healthcare, and dominated both the public and private markets. This era was enabled by an API boom that allowed you to integrate everything.
In the late 2010s and early 2020s, we saw the rise of hyper-specialized tools — for example, webinar hosting software — that were enabled by cheap and flexible cloud computing and the open-source software revolution.
In each of these revolutions, the cost of software development got cheaper, and we moved from one expensive single-vendor product to a smorgasbord of clunkily integrated point solutions. One thing remained the same: As a company, you were buying software, and the main job of IT teams was to integrate these disparate solutions.
GenAI is fundamentally different: You won’t get a ton of value if all you do is buy a software license. Sure, if you get everyone on your team ChatGPT Enterprise and train them on how to use it (which only about 6% of companies have done), you’ll get some productivity gains. But if you want to realize the true value of GenAI, you likely need to build custom products based on proprietary data that give you a critical competitive edge.
But here comes the challenge: Most companies’ product and engineering teams aren’t accustomed to building new products 0-1. To oversimplify things: If you work on the IT side at most mid-market and enterprise companies, your job isn’t to build new products; it’s to make sure that all of the software you’ve bought works well together. Building and launching new products often requires an entirely different skill set and mindset amongst your workforce.
Even legacy tech companies that have built a product in the past face this challenge. Over the past two years, we’ve worked with hundreds of companies to deploy new GenAI solutions. One of the most eye-opening revelations is that they haven’t built a new product in over a decade; they’ve been in maintenance mode, and the folks who built the initial product 0-1 are long gone.
GenAI innovation is fundamentally a talent problem. Most companies’ workforces aren’t designed to build new products zero to one or make the kind of sharp turns that GenAI requires. BCG found that 62% of corporate leaders said a lack of talent was their biggest barrier to AI adoption. In an Adecco survey of 2,000 C-suite executives, 66% said they will need to acquire that AI talent externally rather than developing it internally.
Why Traditional Models Are Failing
When innovation leaders look to the tried-and-true methods of infusing their team with the AI talent they need, they're likely to walk away unsatisfied. Here's why:
The Traditional Hiring Trap
Most organizations' first instinct is to solve this talent shortage the usual way: post some jobs and start recruiting. But this comes with serious challenges:
- Competing for top AI talent with Silicon Valley on compensation and prestige is hard
- Hiring cycles are already long and challenging. In our survey of 581 tech executives, 62% said that it takes four months or more on average to hire top engineering talent, and 67% said that the traditional hiring process is broken and needs an overhaul
- Attracting top talent becomes increasingly difficult when company leadership struggles to present a clear vision; 57% of C-suite executives say they lack confidence in their leadership team's AI skills and knowledge
The Consulting Conundrum
The other common approach is to contract an IT Services firm such as McKinsey or Deloitte. This path, too, comes with serious challenges:
- Traditional IT Services are often ill-equipped for the challenge of generative AI, which at its core necessitates building and iterating on new products the way a startup would
- In a survey of corporate managers who worked with the Big Three consultancies on digital transformation efforts, 84% felt they were "no help at all"
- This model is expensive by nature, making it difficult at a stage when the ROI of new GenAI initiatives isn't clear until you've built a prototype.
A New Model Emerges
But there's hope. Forward-thinking innovation leaders are discovering a powerful alternative: the fractional economy. By tapping into a growing pool of elite tech talent who've chosen independence over traditional employment, companies are building "blended teams" that combine the best of both worlds – the stability of full-time employees with the specialized expertise of independent talent.
This is a modified excerpt from our Blended Teams Playbook. To get the full guide to how innovation leaders are bridging the AI talent gap, download the full report here.