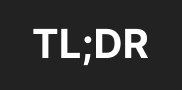
Make sure your AI integration is solving a real problem—not just pursuing AI at all costs.
Begin with managed cloud services and APIs to simplify deployment, and iterate on the product before optimizing.
Avoid long-term commitments and design for flexibility to adapt to the fast-changing AI landscape.
AI has finally come of age. After a cycle of winters and summers, often with flopped, over-hyped promises, the current generation of AI solutions, particularly in the field of generative AI, is delivering stunning results. The most salient case is, of course, ChatGPT, the faster growing product ever, with 100 million users in its first month.
As Kevin Roose put it in the New York Times, "ChatGPT was a moment when a technology people had heard about finally became real to them."
The field is moving at breakneck speed and it's virtually impossible to simply keep up with the news, let alone actual utilize all these new AI tools.
When considering how to incorporate these technologies into your startup, you may feel naturally overwhelmed and confused. Beyond the flashy demo you just saw, what is the impact on your deployment costs? What should your latency requirements be and can they be met by this solution? What about data privacy or legal risks? There's simply a lot to consider, and it'd be only normal to be caught up in analysis paralysis.
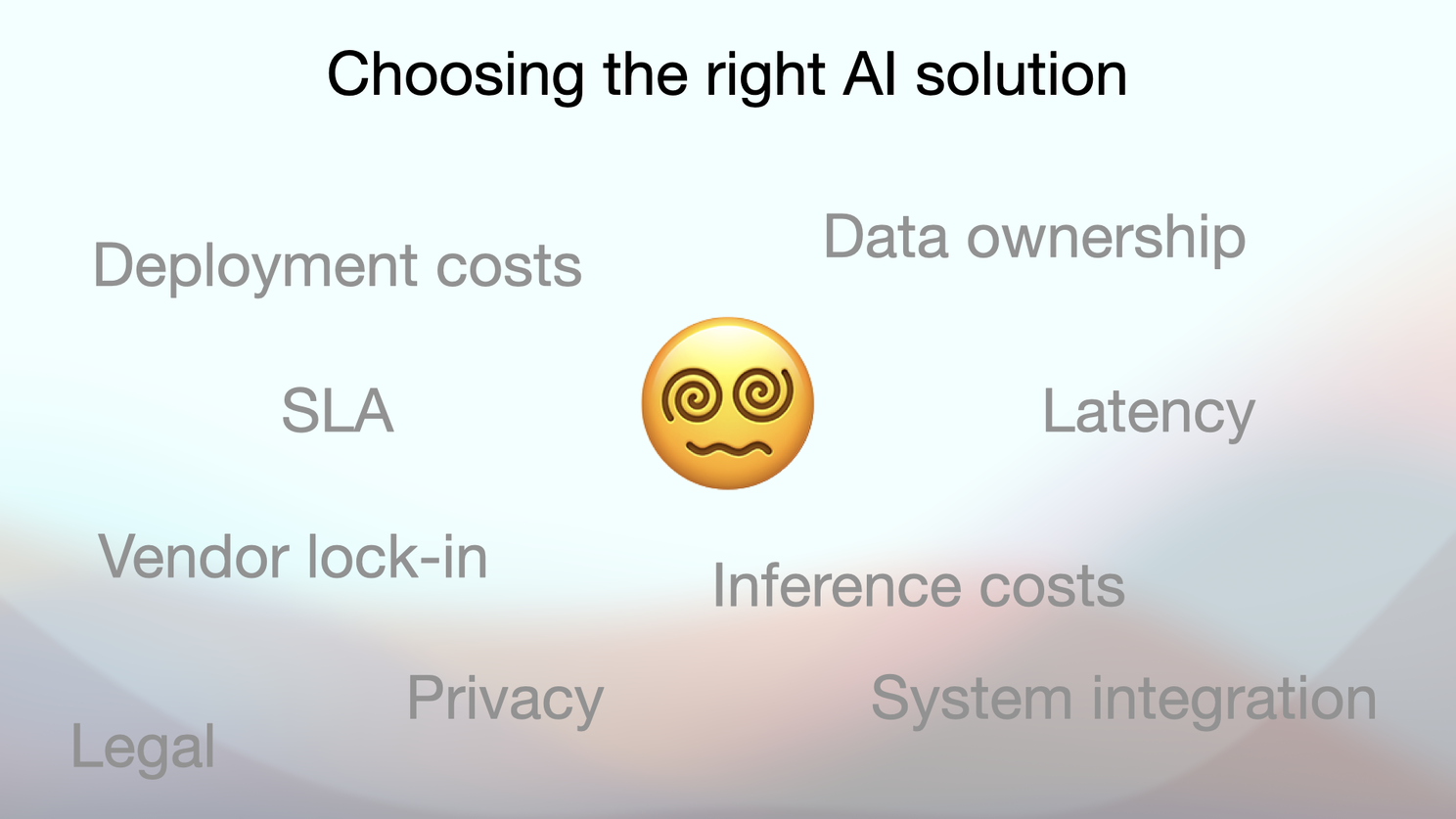
You need a plan.
In this article, I am going to suggest 5 recommendations to kick you into action with confidence.
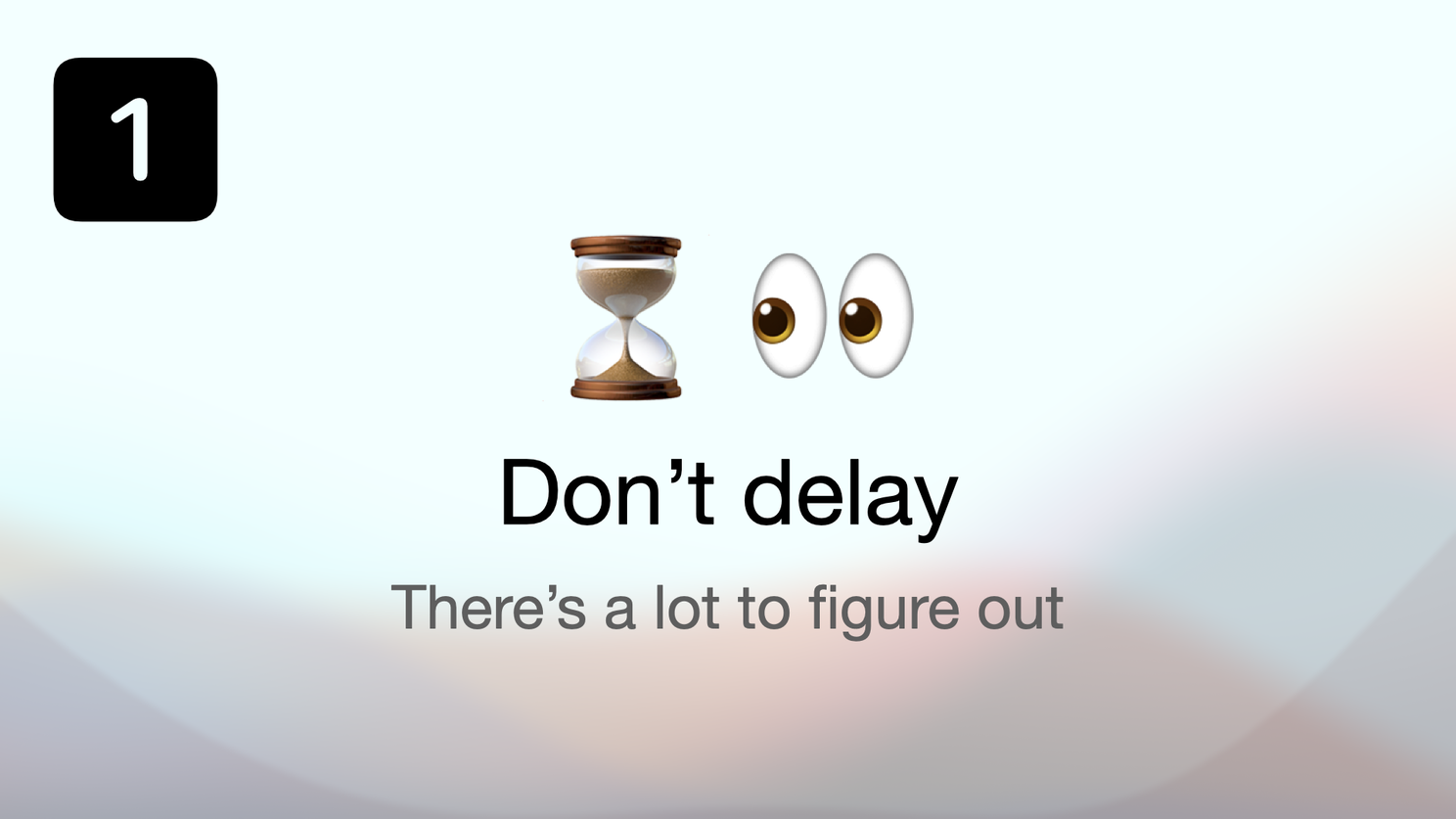
The first recommendation is to not delay—not necessarily because you want to be faster than your competitors, but because there is a lot to figure out in this new and fast changing landscape. For one, you need to think hard about how to achieve product-market fit in this new reality.
For instance, Elon Salfati has built an AI-powered system for cold outreach that feels deeply personalized. He manages that at scale by leveraging OpenAI APIs. Such approaches are going to completely redefine the standard practice in their respective business domains. Likewise, you need to figure out what disrupting use cases will be applied to your business, so that you can stay competitive.
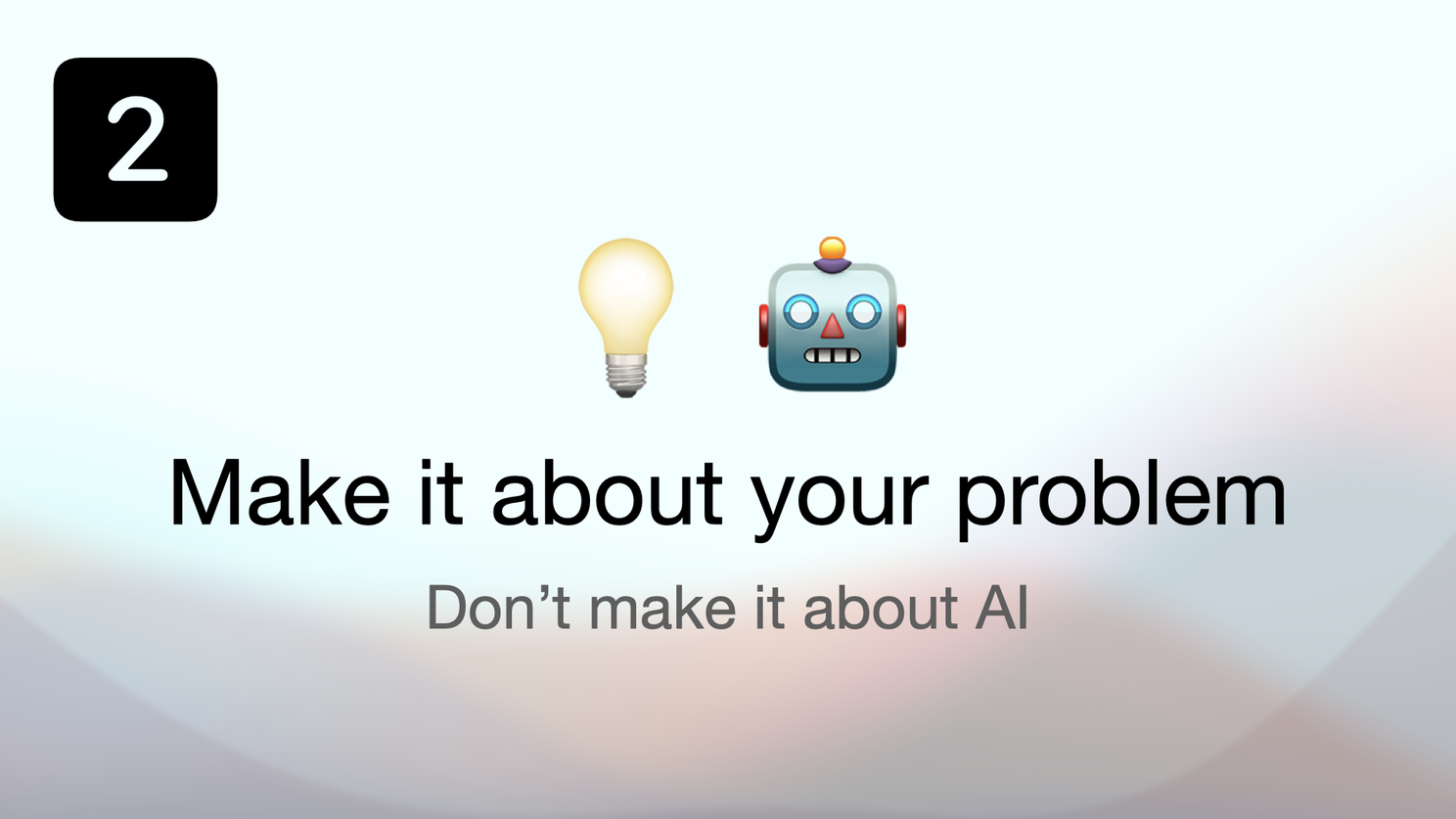
The second recommendation is to make sure you're solving a real problem, not just adding AI at all costs. After all, AI is just a tool, a truly powerful one for sure, but not an end in itself when it comes to use cases. It's easy to fall prey to FOMO and jump into wild adoption without a clear vision. Instead, you can double down on your true super powers: finding product differentiation and iterating on your user testing, until finding the right experience for your customers, in whatever shape and form that happens to be.
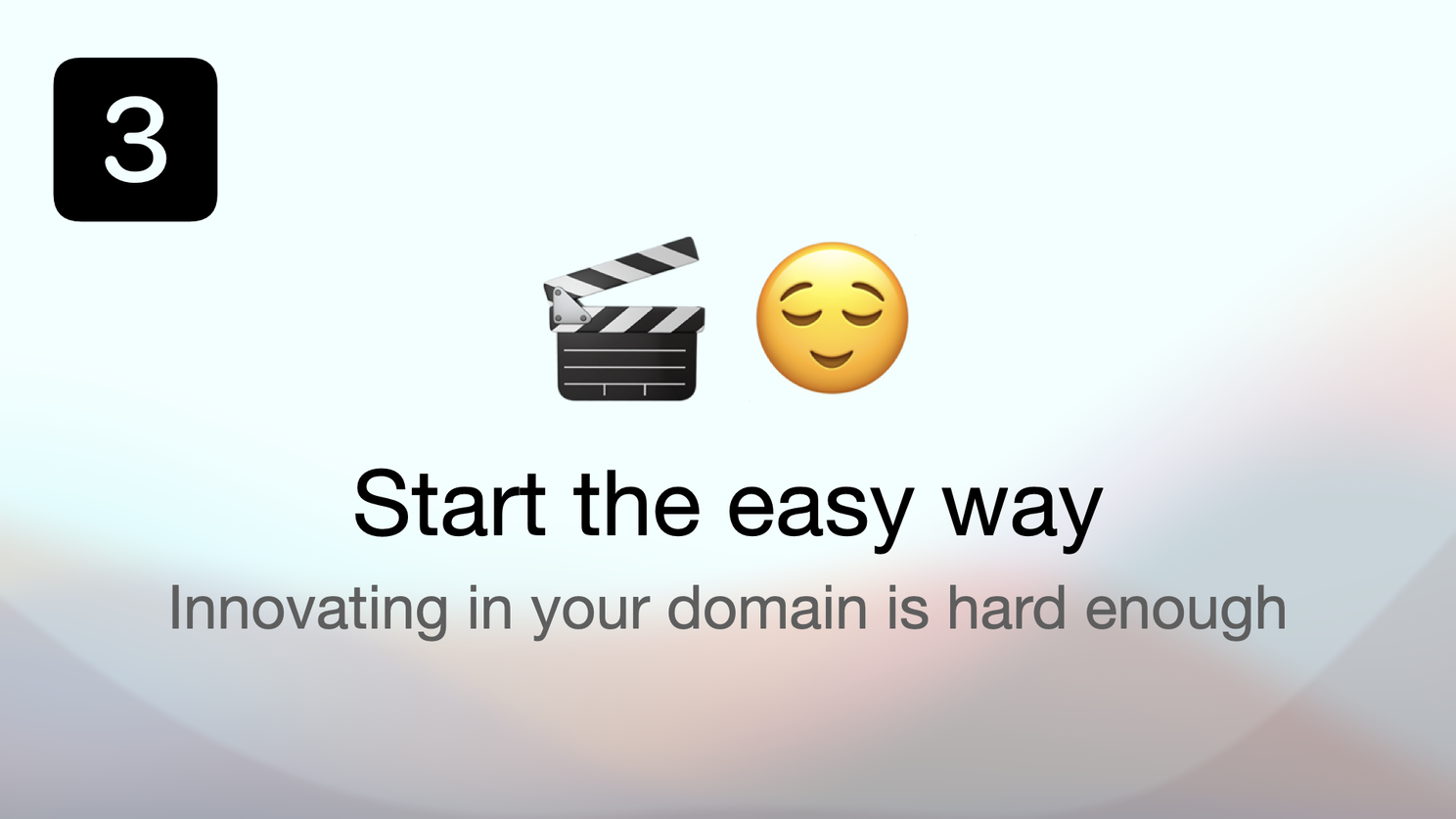
The third recommendation is to start the easy way. There's no need to complicate your life with deployment options, complex inference costs considerations, and latency requirements, when you are still uncertain about how well this new wave of AI solutions will perform on your problem, with your data. I recommend getting started with fully managed cloud services served via APIs. Typically, this would be your OpenAI API, which can be easily integrated into your current system.
For instance, I recently joined a team of four, with roles ranging from front-end developer, to product manager, data engineer, and backend engineer, coordinated by an Innovation Advisor, to put together a compelling generative AI prototype in a weekend hackathon organized by A.Team. We had never worked together before, but we gelled immediately and went from brainstorming, to design wireframes, to prototype, to demo in just 2 days. These technologies are truly bicycles for the mind.
However, you will need to iterate on your product quite a bit at this stage, because it's hard to go from prototype to a production-ready feature with AI. But assuming you have a clear understanding of your customers and a working knowledge of the technology, you will manage. Once you have reached this stage, you are now an AI-powered startup, so celebrate!
While this may feel like an exhilarating moment and it can surely start to unlock previously unavailable efficiencies or capabilities, this won't cut it long-term. The reason for this is that there is no moat, not even for the big players such as Google. Everyone who puts in the time and the effort will eventually get to this phase, and this kind of adoption is only getting easier and easier.
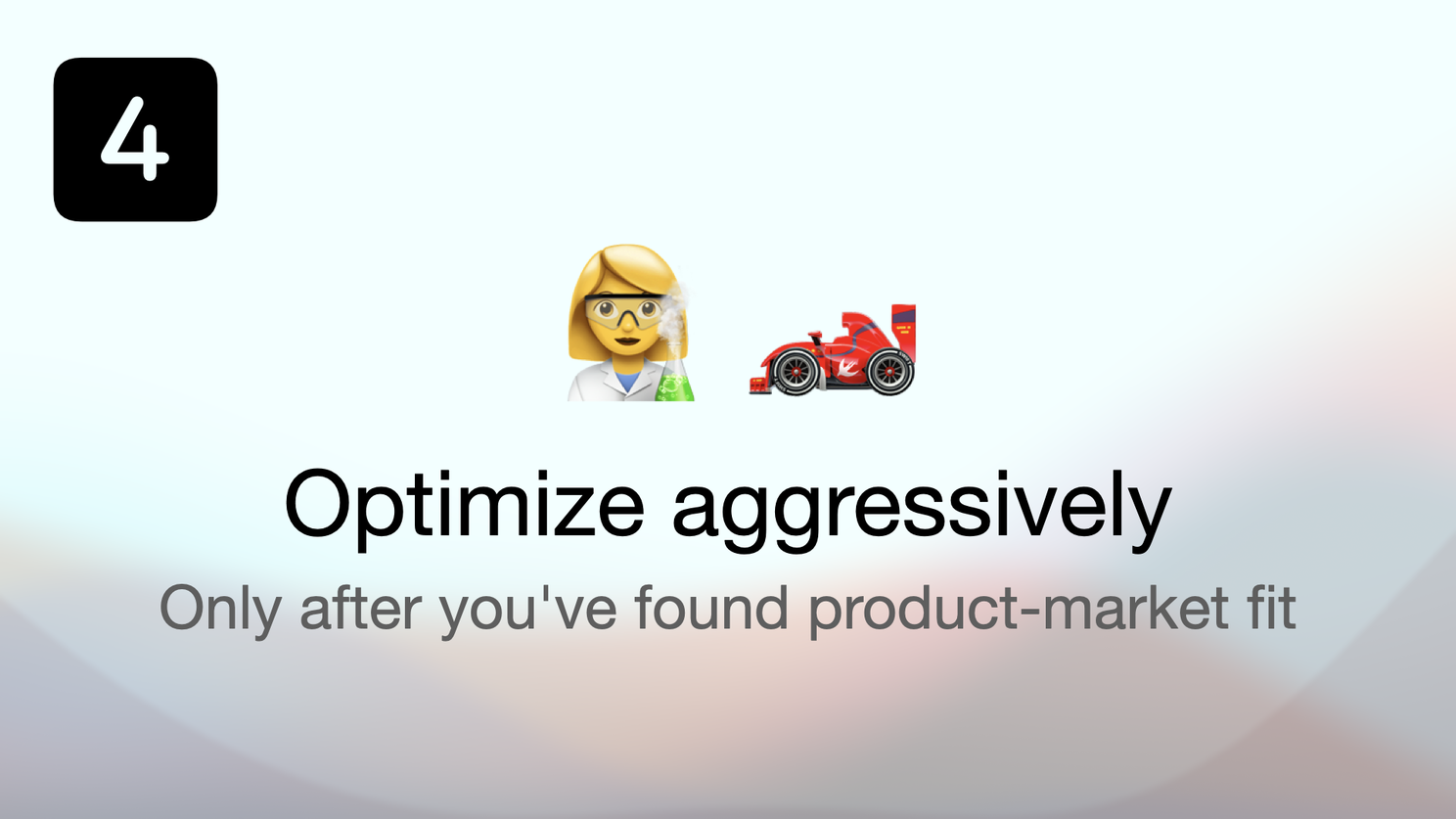
This takes us to recommendation number four, which is to optimize aggressively, once you have figured out product-market fit. This is where you get to flex your engineering muscle and dive deep into model selection and deployment. I argue that there are a ton of opportunities for switching to open source models, which are generally less capable than the flagship models from the big players such as OpenAI, Google, or Anthropic, but that offer unparalleled control. Through careful engineering and model steering, you can achieve a competitive advantage.
Another area where you can rake mounting efficiencies both in cost and latency is in deployment—by going to a self-managed solution. By encapsulating your dependencies in a custom container or by leveraging some off-the-shelf offering, you can easily switch deployment vendors, and trade the likes of Azure or AWS for new players disrupting this space, such as OctoML.
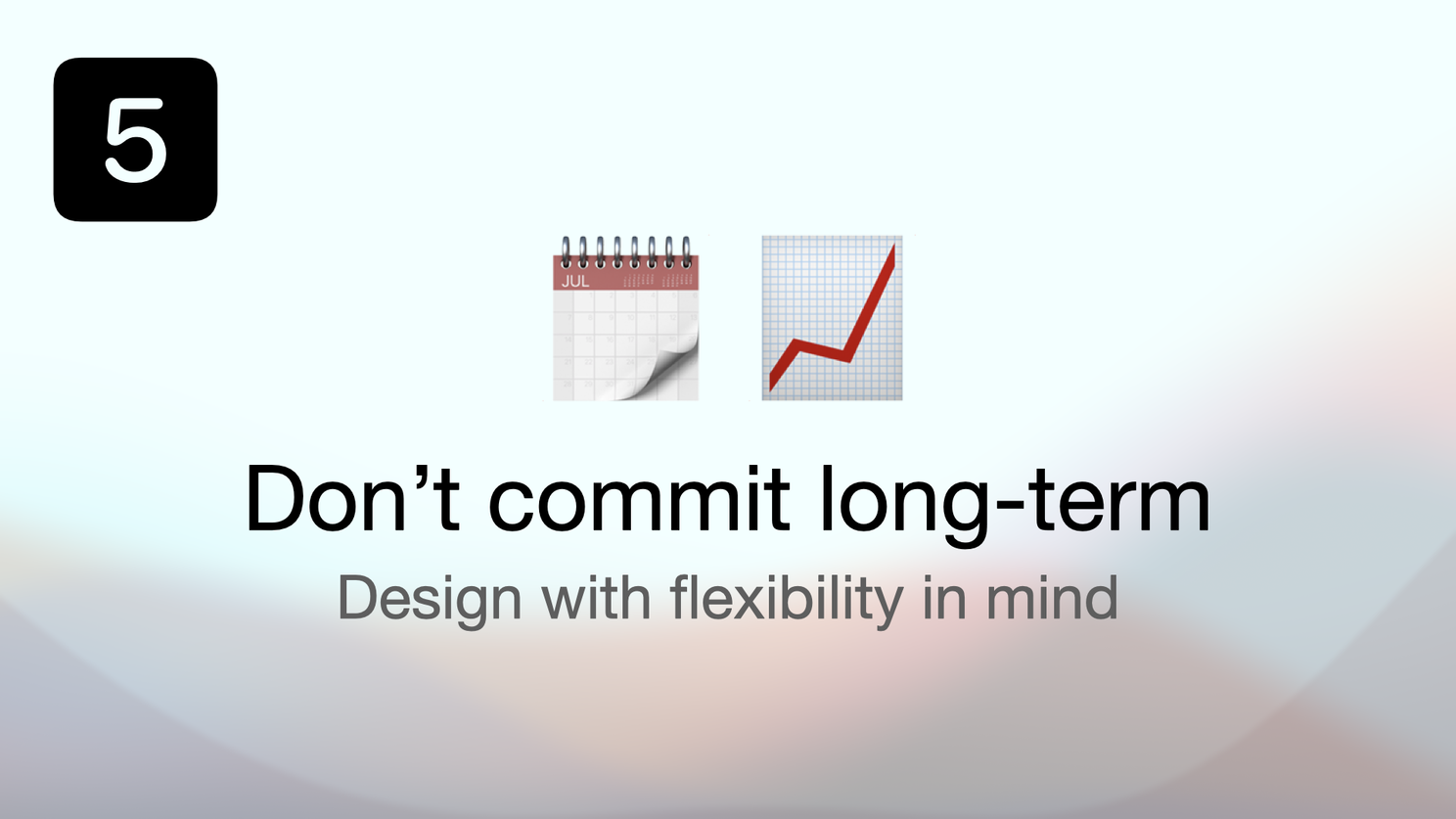
The fifth and final recommendation is to not commit long-term to any vendor contract or even a particular model. The field is changing way too fast and you want to maximize for flexibility.
Take for instance the eruption of falcon, the open-source LLM from Technology Innovation Institute. It took the world by storm by dethroning LLaMA from the HuggingFace leaderboard, initially with a commercially permissive license with a 10% royalty, only to drop the royalty in just 2 days! Such changes can completely turn the tables with regards to what is the most suitable direction for your startup. If you did your engineering right, as described above, you would be in the position to easily switch models (ideally with a drop-in replacement by encapsulating abstractions via libraries such as LangChain).
Here is a recap of all five recommendations:
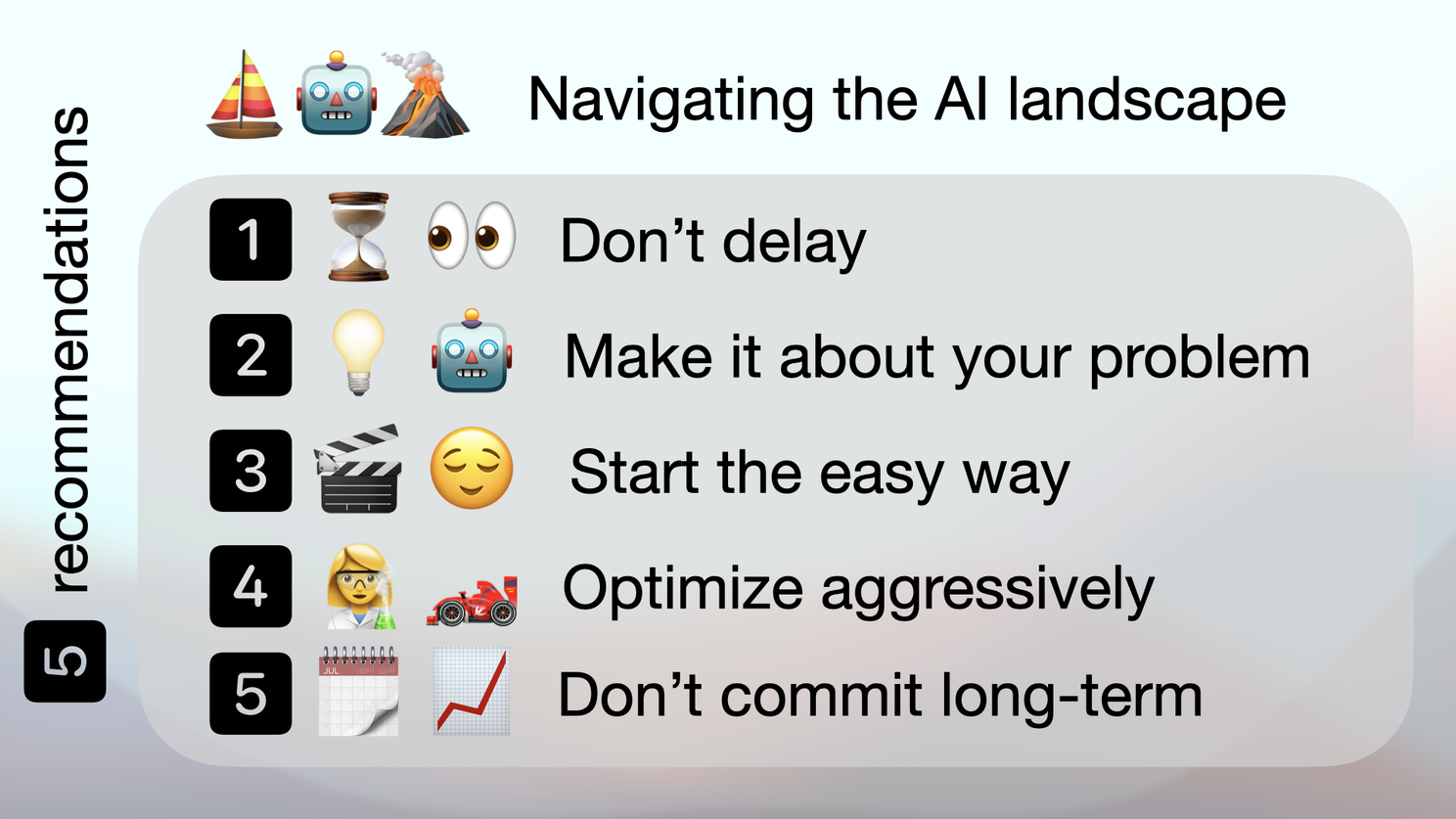
In conclusion, startups looking to incorporate AI need a plan to navigate this new landscape, focusing on their actual problems and customers rather than flashy technologies, starting simple with cloud APIs before optimizing aggressively and avoiding long-term vendor lock-in. With the right approach, AI can be a powerful tool, but it requires diligence and flexibility to utilize without being overwhelmed or left behind.
Adrian Tineo is a Ph.D. in Computer Science and a seasoned software engineer on the A.Team network, where he is also an AI Expert Guild leader. His work in AI ranges from 3D image segmentation in a land surveying application to sports analytics powered by Computer Vision. He leads a small community of AI enthusiasts in Málaga.